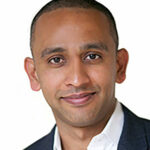
“When the going gets tough, the tough get going”
By Ram Aiyar, CEO of Korro Bio, as part of the From the Trenches feature of LifeSciVC.
It has been quite a start to the 2025 year. The sentiment around biotech starting from the yearly JPM conference has been “sour” at best – see post from Atlas’ Aimee Raleigh (here). Since then, sentiment has gotten progressively worse due to the many uncertainties that exist for investors. When you then layer on the opportunity costs for non-healthcare specialists for return-on-investment, the uncertainty around tariffs, the hushed whispering of the “R” word, the areas where Limited Partners (LPs) look to put their money, all point to not having certainty in the near term.
One thing I am confident in is that, over the next two to five years, companies that will have outsized returns for investors are the ones that are newly formed with appropriate valuations, meet milestones as they are capital efficient, and are focused on developing real product candidates. It is a waiting game through this time of maximal pain (credit to the investor who coined this, who shall not be named). As one of my mentors Peter Bugelski told me when I started at Centocor/J&J: Develop medicines that solve a meaningful medical need, the money will follow.
So, what can leadership teams do to prepare themselves for this time of uncertainty. Below are some thoughts that I have learned through the many startups I have been part off:
- Focus on products that can have meaningful value inflection points
- Consider additional valuing creating elements that build differentiation
- Ensure sufficient runway to provide meaningful updates
- Develop partnering options on all assets to extend runway
- Communicate with all the stakeholders (including family)
- Execute, execute, execute
These themes are constant across all the startups I have been involved with including Korro Bio (President and CEO, NASDAQ listed under KRRO), Triveni Bio (Director), Protean Bio (Director), Corvidia Therapeutics (acquired by Novo Nordisk), Benevir (acquired by J&J), Avidea (acquired by Barinthus). I will walk through moments during my time at Corvidia Therapeutics that highlight some of these elements.
Prior to Korro, I co-founded a company called Corvidia Therapeutics, with three other amazing individuals. The company was formed on the foundation that inflammation (specifically IL-6 mediated inflammation) was the residual risk factor for cardiovascular disease in patients with chronic kidney disease both on dialysis and not-on dialysis. We had the genetics, the endo-phenotypes as well as epidemiological data that demonstrated the potential for outsized benefit specifically in dialysis patients. We also showed that there was a correlation with erythropoietin (EPO) resistance, cardiovascular mortality, and IL-6 levels. We raised $26M, in-licensed a long acting anti-IL6 mAb from Medimmune / AstraZeneca, hired four other individuals. As a team of eight and few consultants, we took the asset from pre-IND through two clinical studies in two different CKD populations and in the dialysis patient populations demonstrated an impact in EPO resistance, with a potential path for approval. Around the time we were ready to raise a Series B (we raised $65M), a big pharma had demonstrated in a large outcome study that our hypothesis was accurate, and our therapy had the potential to have an outsized cardiovascular benefit in the patient population we were targeting.
We were in a conundrum where the potential for the drug to work in a large population was evident, however, as a small company of fourteen individuals (post Series B) we needed an additional $400M to prosecute the cardiovascular outcome study, which would be the definitive Phase 3 study for approval. This is daunting even for a large / mid-size company, let alone a fourteen person one. We knew we had a drug that works. We set out to build optionality along the following verticals
- Could Pharma be interested in partnering?
- Ran a 200+ patient study to show safety that Pharma needed to see
- Developed an auto-injector plan so we can run an outcome study with the right form of delivery
- Communicated with potential partners and key opinion leaders
- Would investors be willing to build a position to take us public?
- Obtained a term sheet from a well-known healthcare specialist
- Extended runway through commitment from our amazing board and investor group
- Reduced capital allocation on additional programs to focus on the lead asset
- Could we find alternate financing models to run an outcome study?
- Secured a term sheet from a project financing entity for ~$350M
- Secured a reverse merger option with an amazing company to merge assets (that company eventually sold for $3B+)
- Could we partner the additional assets we had to increase runway?
- Secured interest on a regional partnership for the lead asset
- Secured interest on our second asset for a discovery deal
We had an amazing team (all fourteen), great investors, and a good board that supported the building of this optionality in a short period (twelve – eighteen months), especially as we entered the pandemic in early 2020. Eventually, due to the strength of the data, the opportunity, and aligned vision, Corvidia was purchased by Novo Nordisk in 2020. The drug (ziltivekimab) is in the right hands!
As you look at the above list of items to pursue, one could say let’s focus only on a few items with the highest likelihood of success. In times of survival, like the one we are in right now, one doesn’t have the luxury of choice. You need the energy, passion and tenacity to pursue all the options on the table. That takes a team, sleepless nights, and ensuring that you are working on the right things with intensity. Communication is essential with not just your stakeholders at the company, but also the ones at home to build alignment.
In conclusion, luck favors the courageous and prepared. Be prepared for everything, ensure survival by working on meaningful programs, and keep all options on the table. Bon Chance. .